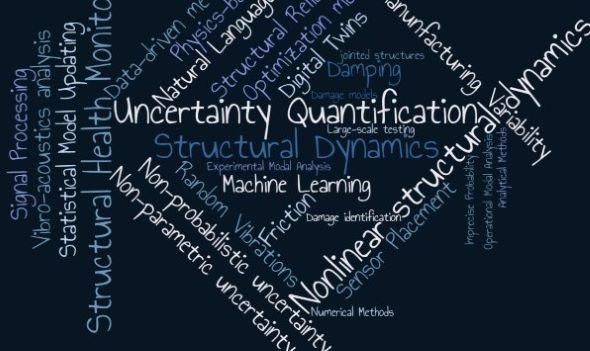
Submitted by Dr Fiorella Del... on Thu, 30/11/2023 - 17:17
In a recent talk, Dr. Alice Cicirello, Assistant Professor in Applied Mechanics, Department of Engineering, University of Cambridge founder and the Head of the Data, Vibration and Uncertainty (DVU) Group, highlighted the critical need for the development of physics-enhanced Machine Learning Algorithms (MLAs) to address challenges in monitoring and forecasting.
Dr. Cicirello's talk explored the overcoming issues such as poor generalization performance and physically inconsistent or implausible predictions that can arise in conventional machine learning approaches and centered around the incorporation of physics-based principles into machine learning algorithms to enhance their performance and reliability. She identified four key biases—observational, inductive, learning, and model form/discrepancy biases—that can be introduced in various machine learning architectures. In her research group, these physics-enhanced architectures play a pivotal role in tackling five specific challenges, with a focus on areas such as friction identification and virtual sensing within engineering structures.
The talk provided insights into how the DVU Group has successfully integrated physics-based knowledge into machine learning frameworks, resulting in more robust and accurate predictions. By addressing biases and incorporating domain-specific information, these algorithms demonstrate improved performance in real-world applications, particularly in structural health monitoring.
Key challenges discussed during the presentation included the identification of frictional forces within engineering systems and the development of virtual sensing techniques. Dr. Cicirello emphasised the importance of bridging the gap between physics and machine learning to create algorithms that not only learn from data but also adhere to physical principles, ensuring more reliable and interpretable outcomes.
In all, the talk underscored the significance of combining domain expertise with machine learning methodologies, heralding a new era in the development of robust algorithms for structural health monitoring and forecasting.
Watch the talk here.