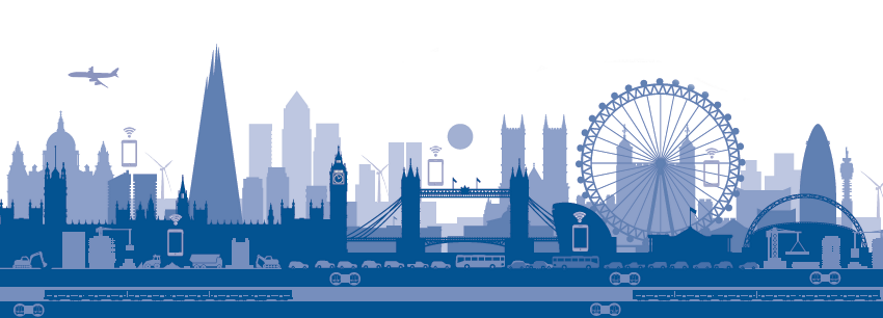
Urban transport networks are under great strain from rising travel demand in areas of rapid population increase. For cities with established transport networks, strict emissions targets and limited physical space for development, it is no longer economically viable or sustainable to deal with increased demand by simply adding new capacity. These case studies show how CSIC’s research – from complex city-scale systems and operations to individual assets – combine to deliver integrated smart solutions for the design and management of UK infrastructure.
Harvesting spatially and temporally dynamic data on travellers
Gerard Casey, Doctoral Researcher
Historically, transport planners have made use of top-down macroeconomic models that treat transport modes as distinct using models informed by static averaged inputs from standardised functions or limited survey data. Computational advances that enable complex, bottom-up fine resolution simulations to be carried out over long time frames, plus the emerging network of geospatially-connected devices, enables the harvesting of fine resolution spatial and temporal data on travellers. Even infrastructure itself permits crowd-sourced data to be used to inform dynamic models with real-world and real-time data. This step-change bypasses the need for generalised functions and/or expensive survey data and informs a framework which consists of a multi-modal agent based model (ABM) that can be used for a range of scenario testing exercises, including hard infrastructure and/or policy changes. The ABM facilitates scaling and makes use of a large repository of spatially and temporally dynamic data in order to provide real-world inputs. It also allows for the macro and micro impacts of changes to be assessed. This novel framework offers a wide range of valuable applications including, for example, how high speed rail usage has evolved in the case of HS1 in the UK and what implications this has for low carbon international travel in this region of Europe.
Understanding travel behaviour in the age of big data
Tim Hillel, Doctoral Researcher
Managing travel demand directly by combining network improvements with policy and regulatory changes, including new scheduling and pricing schemes which encourage passengers to adapt their travel behaviour, brings benefits to operators. This new approach necessitates a significantly deeper understanding of the seemingly random variations of passenger flows. The recent adoption of several notable technologies, including contactless payment cards, mobile phone-based location services, vehicle tracking cameras, and connected vehicles has enabled a step-change in the availability of passenger movement data. Using London as a case study, this project is deploying machine learning techniques to infer causal relationships between network conditions and passenger transport decisions. This is achieved using an iterative loop between deep learning as a pathfinder, and structured, consumer utility-based travel behaviour models as verification. This enables a novel perspective in understanding how people interact with transport services and the wider urban environment, and establishes a new paradigm in travel behaviour modelling in the age of big data.
City-scale infrastructure simulation
Bingyu Zhao, Doctoral Researcher
Traditional degradation models for transport infrastructure focus on the behaviour of discrete elements of a transport network – a single road or railway section – which limits the development of more efficient network-level maintenance plans. New systems-based models are required to increase the efficiency of maintenance programmes and reduce costs. A novel, cell-based system-level infrastructure degradation and maintenance simulation model that interacts with the current abundance of digital information available for infrastructure networks is in development. This new model proposes upscaling traffic infrastructure degradation models to a system (city) scale to enable asset managers to make better informed network maintenance decisions which are optimised for the whole system. This city-scale model uses extensive data obtained from automated surveys covering large spatial scale and many types of structures. It operates on a multimodal transport system and includes condition-influencing factors such as traffic, climate and geotechnical properties. The model symbiotically connects infrastructure to the people using it; more people results in further degradation which may also affect people’s usage of the infrastructure, as well as fuel consumption and emission. Potential benefits from a city-scale model include more effective and efficient use of maintenance budgets and an improved public transport system. This city-scale infrastructure system could potentially transfer to other markets including pipelines and electricity grids.
Using new sensing technology to understand the complex structural response of masonry arch bridges
Dr Sinan Açıkgöz, Research Associate Dr Matthew DeJong, Senior Lecturer in Structural Engineering
Working with Industry Partner Network Rail, CSIC monitored an operational Victorian viaduct in Leeds, one of around 40,000 masonry arch bridges that form an integral part of the UK’s road, rail and waterway infrastructure. The objective was to better understand the dynamic response of the viaduct to rail traffic and identify the mechanisms that drive its degradation, to inform maintenance and long-term management. The research has provided new insights into the behaviour of this type of ageing structure and the potential for a more accurate condition assessment. The sensing data has provided an explanation of existing damage, identification of critical aspects of the structural response, and better evaluation of the effectiveness of potential intervention techniques.
Predictive maintenance of bridges
Zhenglin Liang, Research Associate
Complex industrial assets such as bridges, power transformers etc., are subject to complex deterioration processes. Understanding such complexities and maintaining such assets is a major challenge. This research has designed a novel approach for optimising condition-based maintenance policies for assets with complex deterioration processes. These policies aim to optimise the inspection and replacement plans for such assets and their constituent components so as to minimise the costs and risks involved, while maintaining performance. Working with a local authority, CSIC assessed the effectiveness of the approach on a road bridge system. At the bridge level, detailed deterioration models were formulated for different components of bridges under different exposure levels. At the system level, the economic dependence and two different levels of structure dependence are considered. The timing of maintenance activities is then optimised for the system based on current predictions for both risk and cost, with a goal of reducing the traffic management cost by combining maintenance activities. The result shows that our developed approach has the potential to reduce the maintenance cost by as much as 10 per cent by using this structural asset management planning toolkit.
Download the full case study here