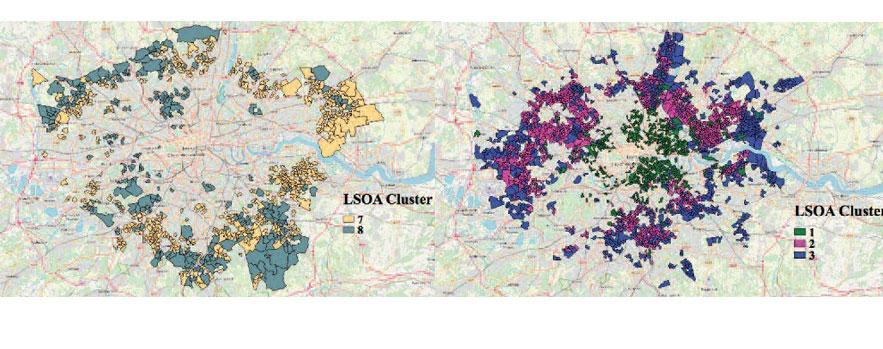
Decarbonising domestic heating
The domestic sector in the UK is responsible for more than a quarter of total energy consumption and greenhouse gas emissions, surpassing all other sectors including road transport and industry 1, 2. Government commitment to reducing carbon dioxide emissions by 80 per cent by 2050 requires effective policy to address domestic energy use.
Decarbonising domestic heating constitutes an essential part of the full path to achieve the 2050 goal, and a range of national government policies and initiatives have been introduced over the years with varying degrees of success. Promotion and implementation of these national policies has fallen to local authorities.
While most cities in the UK have reduced emissions per capita, there is a large variation of domestic gas consumption (the primary fuel used to heat homes in the UK) both within and across cities; compared to the bottom 10 per cent, the top 10 per cent of households consume at least three times more gas 3. Successful implementation of energy policies is only possible if the range of consumer needs and behaviours are understood making knowledge of the nature of these variations an essential step for effective design and implementation of policies that regulate newly built homes and encourage households to improve energy efficiency of existing homes.
Through statistical clustering, we have identified plausible energy demand patterns, and probabilistically grouped households where the same combination of features explain consumption. Unlike regression models, clustering provides a more integrated view by taking all the diverse but relevant information into account at the same time. In cluster analysis, correlated variables only strengthen the identification of patterns (similarities) towards certain directions, which is a reflection of real-world conditions.
Dr Ruchi Choudhary, Data-Centric Engineering Group Leader at The Alan Turing Institute and CSIC Investigator
Contributing factors to gas consumption
Research to date suggests variations are as much a result of the built environment as of wider socio-economic-demographic features of the household but their combined influence on variations of residential heating consumption is not well understood. Exploring the interaction between the numerous contributing factors to the different levels of consumption brings increased insight and additional information that can support local authorities to deliver more efficient policy aligned to securing carbon zero.
A data-driven framework
The UK government, local authorities and public bodies publish a wide-ranging and high-quality collection of datasets available to the public. CSIC researchers are working in collaboration with The Alan Turing Institute to take a data-driven approach to optimise the use of data to support more effective urban energy policy and better decision-making. The data-driven approach also identified similarities between a number of local authorities suggesting the possibility of increasing efficiency by collaborating to share knowledge, promote policy and allocate resources.
CSIC researchers collected all available public data at urban analysis level to gain as much information as possible about domestic heating across different local authority areas in London. Unsupervised machine learning algorithms were applied to create clusters of gas consumption-related information rather than the sole focus being levels of gas consumption. The characteristics of the data at each level were matched to the most suitable clustering algorithm.
A two-step clustering framework was selected because the highest resolution of available data is usually at a different scale from the more aggregated scales at which policies are executed. Residential gas consumption data is available in the UK at the Lower Super Output Area (LSOA) level (uniformly constructed data from the national census). CSIC researchers were able to preserve the more detailed and accurate information contained at this level and aggregate the outcomes to be relevant for understanding variations of gas consumption across different Local Authority Districts (LAD). The two-step framework comprises LSOA-level and LAD policy-level data enabling executive bodies to focus on a range of results according to stakeholder interest. In addition, the framework enables both a numerical and spatial analysis without directly including the target variable and geographical information in the modelling process. The multi-layered approach applies different algorithms to result in more insights into urban energy consumption.
Variables affecting gas consumption
The clustering framework was used to analyse the variations of residential gas consumption across the different LADs. A total of 18 variables related to domestic gas consumption were collated including residential energy consumption, built-environment features, and social-economic and demographic data of the Greater London Area (home ownership; employment status; ages of occupants; health of occupants; median income; and type of house); domestic gas consumption information is not directly included in the clustering process. Except for the built environment and energy- efficient ratings, all other data were collected in 2011. Traditional approaches to analysing variables affecting domestic gas consumption tend to prioritise the quality of the dwelling whereas the inclusion of LSOA data in the framework enables a wider and richer range of socio-demographic and locational constraints affecting residential energy demand to be identified. This framework not only invites comparison between buildings but also between local authority areas.
Eight clusters were generated showing differences in levels of gas consumption (selection shown above). In clusters indicating similar levels of gas consumption the framework identified a range of spatially and numerically different variables suggesting various drivers behind the level of consumption.
Informing policy
Taking a data-driven approach enables domestic gas consumption to be explored in much greater detail. The framework provides an application showing how to work with a range of data and match the machine learning algorithm to urban scale problems which can be adopted by local authorities. Generating richer information about the different drivers behind domestic gas consumption offers executive bodies responsible for implementing policy to be better informed and address specific challenges related to levels of consumption in a more effective manner, and target policy accordingly.
Next step
The framework shows relationships and similarities between local authorities in relation to domestic gas consumption suggesting the possibility of collaboration to share information and make best use of limited resources. The framework could be extended to a nation- wide study of domestic gas consumption in cities and adapted for urban analysis of other topics such as identifying communities that can be transitioned to zero-carbon more easily than others.
Applying this data-driven approach in India
Slipping through the net: targeting energy poverty interventions in India
Lack of access to clean fuels in fast growing cities in India poses risks to health, the environment, and livelihoods, with many households still reliant on solid biomass fuels to meet some or all of their energy needs. Policies to promote the uptake of clean fuels are often national in scope with a focus on cost, and not targeted to the needs of local communities. Modelling of residential energy trends in India, and definitions of energy poverty, have usually been based on income. However, many of the challenges in accessing and adopting cleaner fuels are influenced by a combination of non-income factors.
Households can follow different pathways in transitioning to clean fuels and these pathways feature different challenges and barriers that must be overcome. For example, labourers may struggle to save their daily wages to make the monthly gas cannister payment, whereas households in informal settlements may be unable to benefit from subsidies intended for them due to land tenure issues. Identifying such transition pathways in India is made difficult by data scarcity and lack of appropriate analytical approaches.
CSIC researchers in collaboration with the Indian Institute for Human Settlements (IIHS) in Bangalore, carried out a targeted survey of 2100 low-income households in five cities across the south Indian states of Karnataka, Tamil Nadu, and Kerala. A data- driven approach was used to identify the different clean cooking transition pathways amongst low-income households, combining both socio-economic and demographic data with in-depth interviews. A two step-clustering method allowed for additional information to be gained from the data by cross referencing the findings from the interviews and survey data to reveal patterns not otherwise identifiable.
In Bangalore four different transition pathways were identified, each with its own unique policy challenge. Using these pathway definitions from the rich dataset researchers are developing a model for a decision support tool which can use publicly available data to identify the likely transition pathways in a given ward and highlight the associated policy issues so as to target interventions to address these.
A kitchen of a typical low-income household in Kochi, Kerala, with both a biomass‘chulha’ stove (on right) and a government subsidised dual burner gas stove (on left).
CSIC team: Dr Ruchi Choudhary, Mingda Yuan, André Paul Neto-Bradley
J. Palmer, I. Cooper, United kingdom housing energy fact file 2013, Department of Energy & Climate Change, Prepared under contract to DECC by Cambridge Architectural Research, Eclipse Research Consultants and Cambridge Energy. The views expressed are not necessarily DECC's p172 (2013).
2 BEIS, Energy Consumption in the UK (ECUK) 1970 to 2018, 2019.
3 J. Morley, M. Hazas, The significance of difference: Understanding variation in household energy consumption.